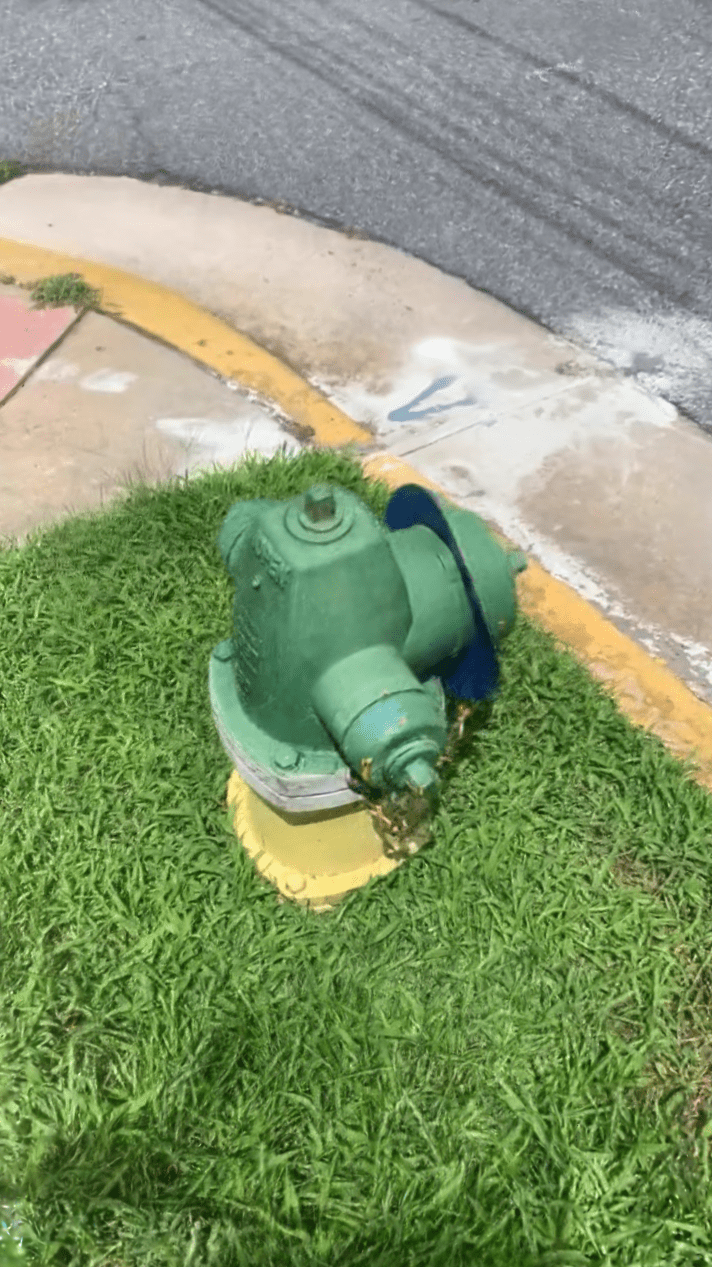
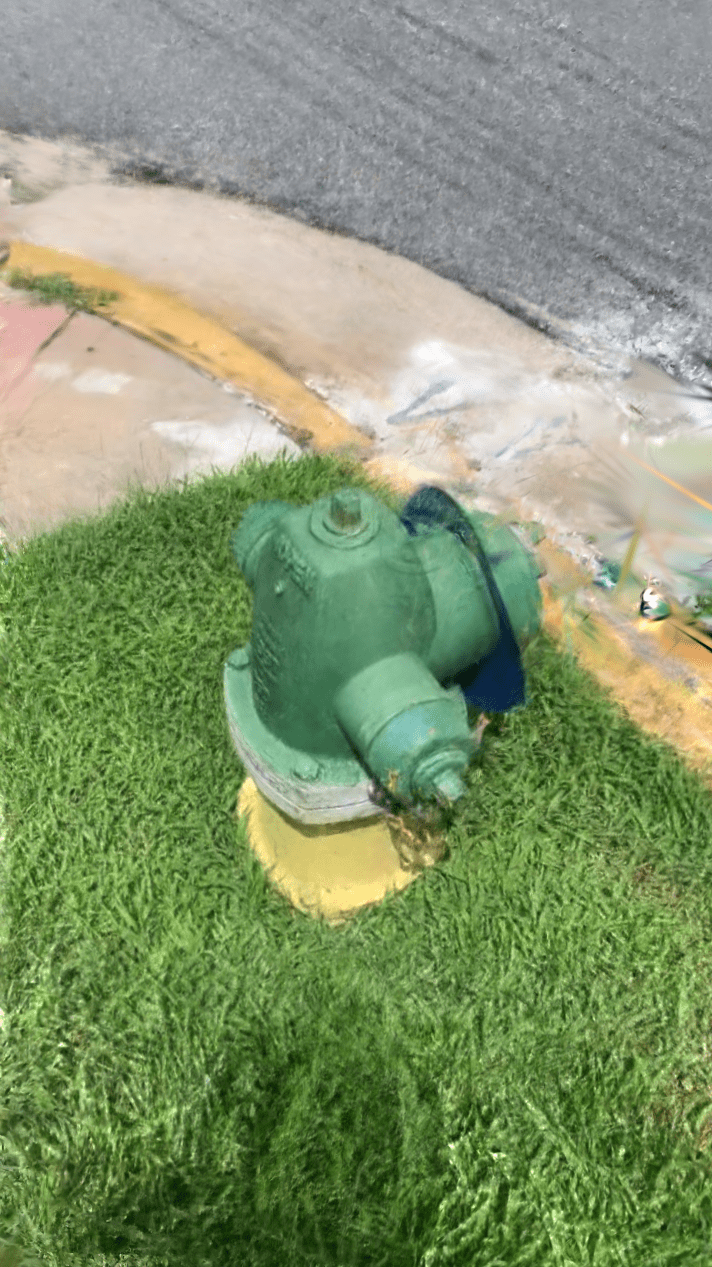
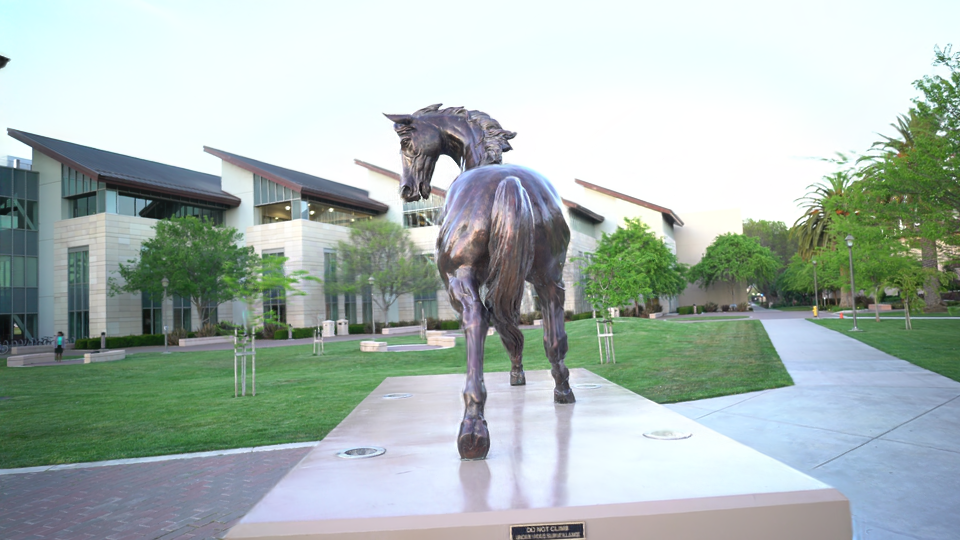
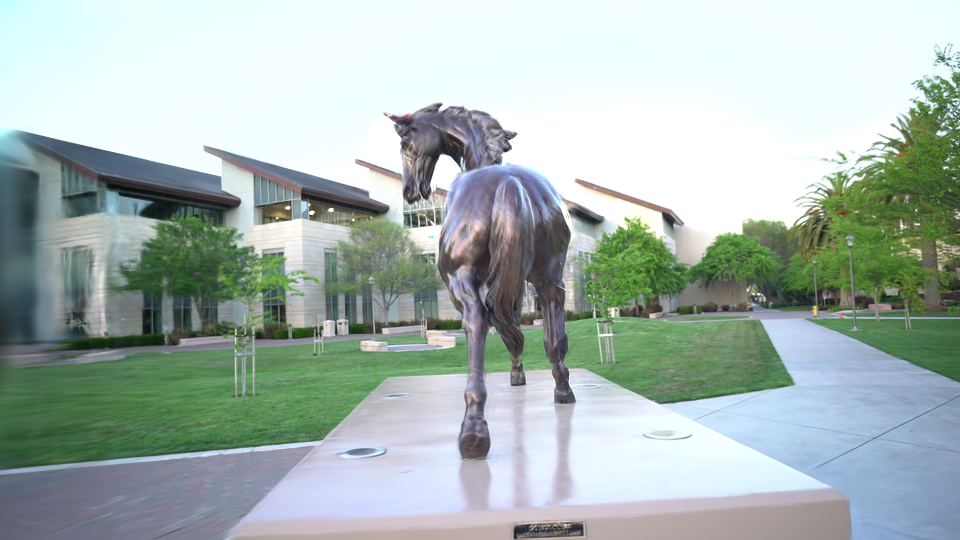
Efficient and accurate 3D reconstruction from monocular video remains a key challenge in computer vision, with significant implications for applications in virtual reality, robotics, and scene understanding. Existing approaches typically require pre-computed camera parameters and frame-by-frame reconstruction pipelines, often leading to error propagation and substantial computational costs.
In contrast, we introduce VideoLifter, a novel method that leverages geometric priors from a learnable model to incrementally optimize a globally sparse to dense 3D representation directly from video sequences. VideoLifter segments the video sequence into local windows, where it matches and registers frames, constructs consistent fragments, and aligns them hierarchically to produce a unified 3D model. By tracking and propagating sparse point correspondences across frames and fragments, VideoLifter incrementally refines camera poses and 3D structure, minimizing reprojection error for improved accuracy and robustness.
This approach significantly accelerates the reconstruction process, reducing training time by over 82% while surpassing current state-of-the-art methods in visual fidelity and computational efficiency.
VideoLifter takes uncalibrated images as input and reconstructs a dense scene representation based on self-supervised photometric signals. It performs efficient sparse reconstruction through the use of ``feature points" to formulate an initial solution for globally aligned camera poses. It subsequently optimizes a set of 3D Gaussians and corrects erroneous estimations from each fragment, hierarchically aligning them into a globally coherent 3D representation.
We show novel views from a Bezier curve which is fitted to estimated camera poses.
We show the synthesized images from testing views.